How AI and Big Data Are Improving built on the analysis of risk. Lenders, whether banks, credit unions, or other financial institutions, have to evaluate borrowers’ ability to repay loans before they approve any credit. Historically, this process relied heavily on traditional metrics such as credit scores, income levels, and employment status. However, as artificial intelligence (AI) and big data technologies continue to advance, they are transforming the way lenders assess loan risk and make credit decisions. By leveraging vast amounts of data, AI can help lenders identify potential risks more accurately and quickly, improving loan decision-making, enhancing customer experience, and reducing defaults.
This article explores how AI and big data are revolutionizing loan risk assessments, the tools and techniques involved, and the significant impact they have on the financial sector.
The Changing Landscape of Loan Risk Assessments
In the past, lenders typically relied on a few traditional indicators, such as FICO scores, debt-to-income ratios, and previous credit history, to assess the creditworthiness of potential borrowers. While these metrics are still valuable, they do not provide a complete picture of an individual’s or business’s financial health. Traditional credit assessments also have limitations, such as excluding individuals without a credit history or those who may have been subject to errors or outdated data.
With the arrival of AI and big data, lenders can now analyze far more granular and comprehensive datasets, which allows for more accurate and dynamic risk assessments. These technologies enable lenders to incorporate a variety of factors beyond the standard credit score, including social media activity, transactional behavior, and even psychometric data, resulting in more nuanced and precise decisions.
How AI and Big Data are Transforming Loan Risk Assessments
AI and big data technologies are making loan risk assessments more accurate, efficient, and scalable. Here are the main ways in which these innovations are improving the loan assessment process:
1. Advanced Predictive Analytics
Predictive analytics, powered by AI, is one of the most significant ways big data is transforming the loan risk assessment process. AI models use historical data and algorithms to identify patterns and trends that may be indicative of future behavior. In loan risk assessments, AI uses this data to predict a borrower’s likelihood of repaying a loan, allowing lenders to better assess potential risks.
For example, an AI model might analyze a borrower’s spending patterns, payment history, employment trajectory, and even social media activity to gauge financial behavior. Unlike traditional methods, which often rely on static data points, predictive analytics can evaluate the trajectory of a borrower’s financial situation over time, providing a more accurate picture of their risk profile.
These models are continuously refined as they process more data, leading to more reliable predictions over time. By using machine learning (a subfield of AI), these algorithms can adapt and improve as new data is introduced, learning from past mistakes and successes.
2. Better Data Utilization
How AI and Big Data Are Improving amount of information generated every day by individuals and businesses. This data comes from various sources, including credit transactions, online activities, social media, mobile apps, and more. In the past, lenders only had access to a small fraction of this data—primarily credit reports and basic demographic information.
Today, lenders can leverage big data analytics to analyze vast amounts of data from multiple sources. These datasets can provide valuable insights into borrowers’ behavior, financial habits, and even their personality traits. For example, a lender may be able to assess a borrower’s financial habits by analyzing transaction data from their bank account or mobile payments. Similarly, AI-powered sentiment analysis tools can analyze online behavior and social media activity, helping lenders assess the borrower’s financial stability and even their level of responsibility in managing credit.
The ability to incorporate diverse data points into risk assessments allows lenders to make more informed and comprehensive decisions. Additionally, it helps reduce bias that may arise from relying solely on traditional credit scores, which may not accurately reflect an individual’s true ability to repay a loan.
3. Real-Time Risk Evaluation
Big data and AI technologies enable real-time analysis of borrowers’ creditworthiness. In the past, loan approval processes could take days or even weeks, as banks and lenders manually reviewed applications and gathered data. Today, AI-driven tools can instantly process loan applications and deliver decisions based on a comprehensive evaluation of the borrower’s risk profile.
For example, AI systems can access up-to-date financial data, evaluate the borrower’s spending habits in real-time, and assess other risk factors, such as income stability or changes in employment. This allows lenders to make quick decisions and potentially approve loans faster, improving the customer experience.
Moreover, AI and big data facilitate continuous monitoring of loan performance throughout the repayment period. Lenders can monitor changes in borrowers’ financial behaviors in real-time and adjust risk assessments accordingly. This proactive approach helps lenders identify early signs of default and take action before a loan goes into delinquency.
4. Customized Loan Offers
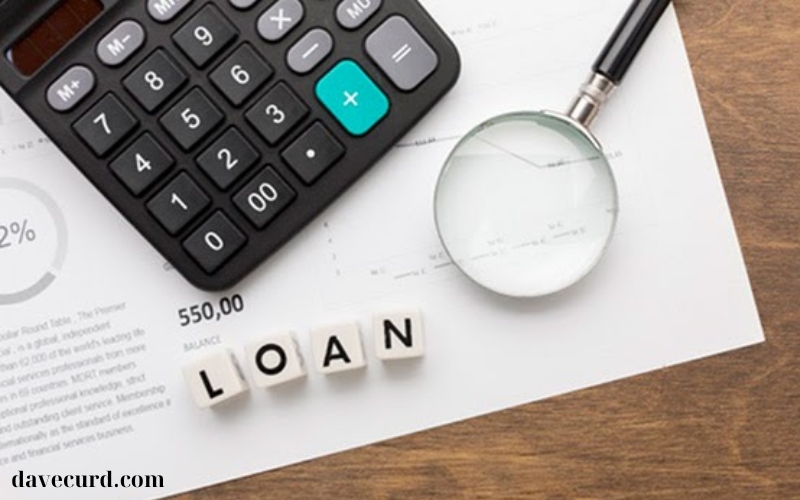
AI-driven risk assessments allow lenders to offer more personalized loan products. Based on a borrower’s unique risk profile, AI can determine the best loan terms, including the interest rate, loan amount, and repayment schedule. This customization ensures that the borrower receives a loan that fits their specific financial needs and risk profile, rather than a generic loan offer based on broad assumptions.
For example, AI algorithms can analyze a borrower’s credit history, income level, and transaction patterns to offer a loan with terms that are better suited to their financial situation. In turn, this reduces the likelihood of loan defaults, as borrowers are more likely to be able to afford the loan repayment terms.
Furthermore, by offering customized loan products, lenders can improve customer satisfaction, as borrowers feel that the loan offer is specifically tailored to their needs and circumstances.
5. Improved Credit Scoring Models
Traditional credit scores are based primarily on factors such as past credit history, debt levels, and payment behaviors. However, these scores may not fully capture the complexity of an individual’s financial situation, especially for people with limited credit history or those who have been subject to past mistakes that do not reflect their current financial behavior.
AI and big data are improving credit scoring models by integrating additional data points into the evaluation process. For example, AI models can take into account non-traditional data such as utility bills, rental payments, and even behavioral data from social media or online activity. By analyzing these alternative data points, AI systems can create a more holistic and accurate view of a borrower’s creditworthiness, especially for those without traditional credit histories.
This shift is particularly beneficial for underbanked or unbanked individuals who may not have a comprehensive credit report. By incorporating alternative data, lenders can extend credit to a wider pool of borrowers, including those who may have been previously excluded from the financial system.
6. Risk Mitigation and Fraud Detection
AI-powered systems are particularly effective at identifying fraudulent activities or potential risks associated with loan applications. By analyzing vast amounts of data in real-time, AI can detect unusual patterns that may indicate fraud or other risky behaviors. For example, AI systems can flag inconsistencies in application data, such as mismatched addresses or conflicting employment details, that may indicate fraudulent intent.
In addition to detecting fraud, AI can also help identify signs of financial distress early on. AI systems can monitor borrowers’ payment history, spending habits, and changes in financial behavior to detect early warning signs of potential loan defaults. By identifying these signs early, lenders can take proactive measures, such as offering payment deferrals or restructuring loans, to reduce the risk of default and mitigate losses.
Benefits of AI and Big Data in Loan Risk Assessments
The integration of AI and big data in loan risk assessments offers numerous benefits for both lenders and borrowers:
1. Enhanced Accuracy and Precision
AI and big data enable more accurate risk assessments by analyzing a broader range of data points, resulting in more informed decision-making. This leads to better loan approval decisions and a reduction in the number of defaults or delinquencies.
2. Faster Decision-Making
AI-powered systems can process loan applications and deliver decisions in real-time, speeding up the approval process and improving the customer experience. Borrowers no longer need to wait days or weeks for loan approval, and lenders can increase operational efficiency by automating the decision-making process.
3. Reduced Bias
Traditional credit scoring models can be biased, as they often rely on limited datasets or demographic factors that may unfairly disadvantage certain groups. AI and big data allow for a more nuanced analysis of borrowers’ risk profiles, reducing bias and providing greater access to credit for underrepresented populations.
4. Improved Customer Experience
With AI and big data, lenders can offer personalized loan terms that better suit individual borrowers’ needs and financial situations. This personalization improves customer satisfaction and fosters long-term relationships between borrowers and lenders.
Challenges and Ethical Considerations
Despite the many advantages, the use of AI and big data in loan risk assessments also comes with challenges. One of the key concerns is privacy. The use of personal data, such as financial transactions, social media activity, and even psychometric data, raises important questions about data security and consumer privacy. It is essential for financial institutions to implement strong data protection measures and comply with regulations such as the General Data Protection Regulation (GDPR).
Another challenge is the potential for algorithmic bias. Although AI can reduce bias in loan assessments, poorly designed algorithms or biased training data can perpetuate discrimination, especially against certain demographic groups. It is crucial for lenders to regularly audit AI models for fairness and transparency.